Advancing Mathematical Modeling with Artificial Intelligence by Bridging Computational Intelligence and Analytical Rigor for Enhanced Predictive Accuracy
Abstract
The integration of Artificial Intelligence (AI) in mathematical modeling has revolutionized computational analysis, enabling more accurate predictions and complex problem-solving across diverse scientific and engineering domains. This paper explores the synergy between AI-driven computational intelligence and traditional analytical methods, highlighting their combined potential to enhance predictive accuracy, optimize decision-making, and improve efficiency in solving nonlinear and high-dimensional mathematical problems. Machine learning algorithms, deep learning architectures, and symbolic AI techniques are examined in their roles of refining mathematical models through adaptive learning, pattern recognition, and real-time data assimilation. Additionally, we address the challenges of interpretability, algorithmic bias, and computational efficiency in AI-assisted mathematical modeling. The study presents case applications in fluid dynamics, financial modeling, biomedical simulations, and climate science, demonstrating the transformative impact of AI in advancing mathematical frameworks. In fluid dynamics, AI-powered models improve turbulence prediction and optimize flow control strategies. Financial modeling benefits from AI’s ability to analyze vast datasets for risk assessment and market trend forecasting. Biomedical applications include AI-driven simulations for disease progression modeling and personalized medicine. Moreover, climate science leverages AI to enhance weather forecasting and climate change prediction. Furthermore, we discuss future research directions, emphasizing the need for hybrid methodologies that integrate AI-driven automation with rigorous mathematical theories to address limitations in interpretability and computational complexity. By bridging computational intelligence with analytical rigor, this study aims to establish a framework for more robust and reliable mathematical modeling solutions in the AI era, fostering innovation across various scientific disciplines.
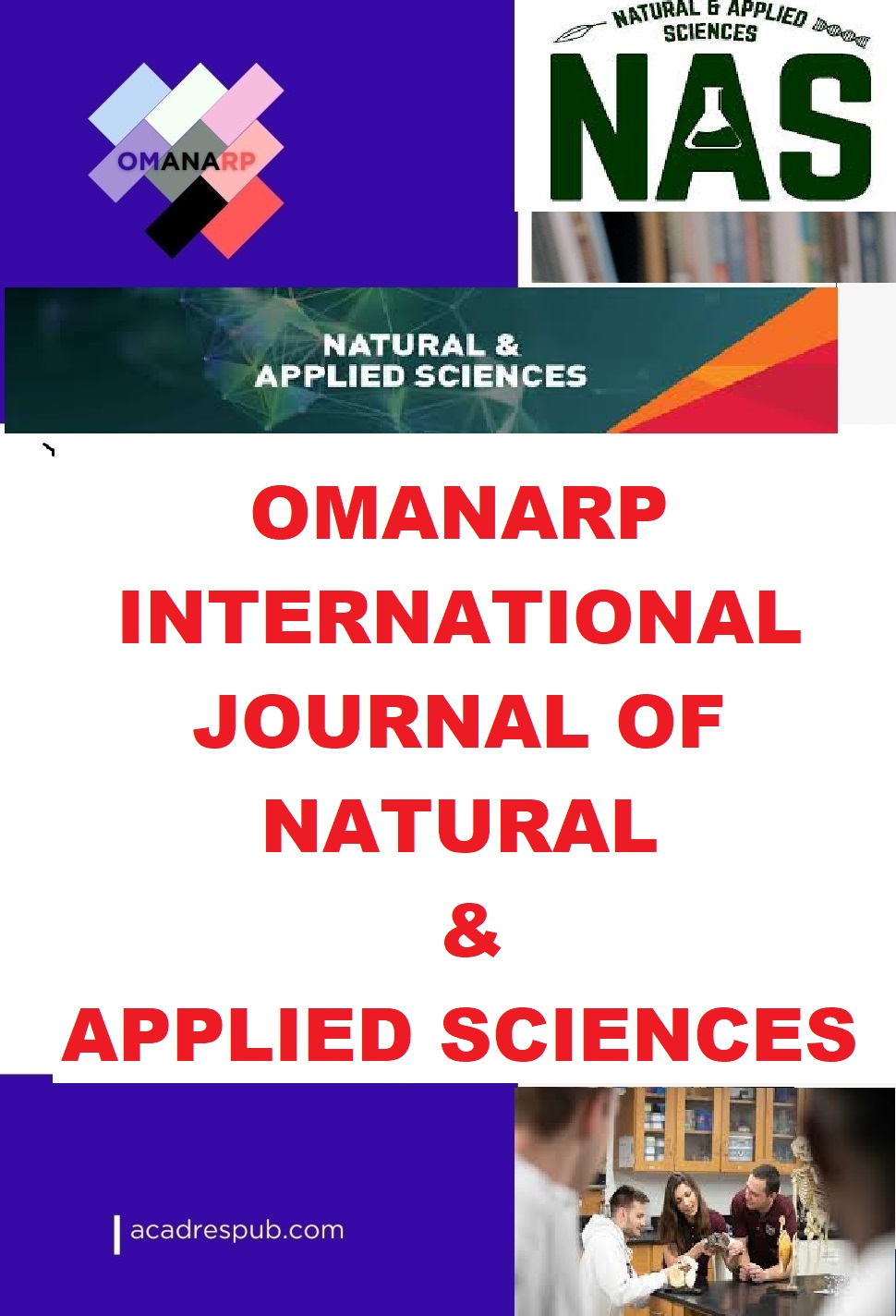