A COMPARTIVE STUDY OF DERIVATIVE- FREE QUASI-NEWTON AND TRUST-REGION METHODS USING FINITE DIFFERENCE APPROXIMATIONS
Derivative-free optimization, finite difference, Quasi-Newton method, Trust-Region method, global convergence
Abstract
This study investigates the implementation and performance of derivative-free optimization (DFO) algorithms for unconstrained problems, focusing on finite difference approximations for gradient and Hessian calculations in Quasi-Newton and Trust-Region frameworks. The motivation arises from practical scenarios where derivatives of objective functions are unavailable or computationally prohibitive. Two methods are proposed: (1) a finite difference-based Quasi-Newton algorithm and (2) a derivative-free Trust-Region method. Numerical experiments on benchmark problems, including the Rosenbrock function, are conducted using Maple. Results demonstrate that both methods achieve global convergence, with the Quasi-Newton method exhibiting faster computational efficiency due to simpler model construction, while the Trust-Region method offers superior accuracy in specific cases. The finite difference approach outperforms traditional quadratic interpolation methods in convergence speed. This work contributes to DFO literature by validating the robustness of finite difference approximations in derivative-free frameworks and providing insights into algorithm selection based on problem complexity and computational constraints.
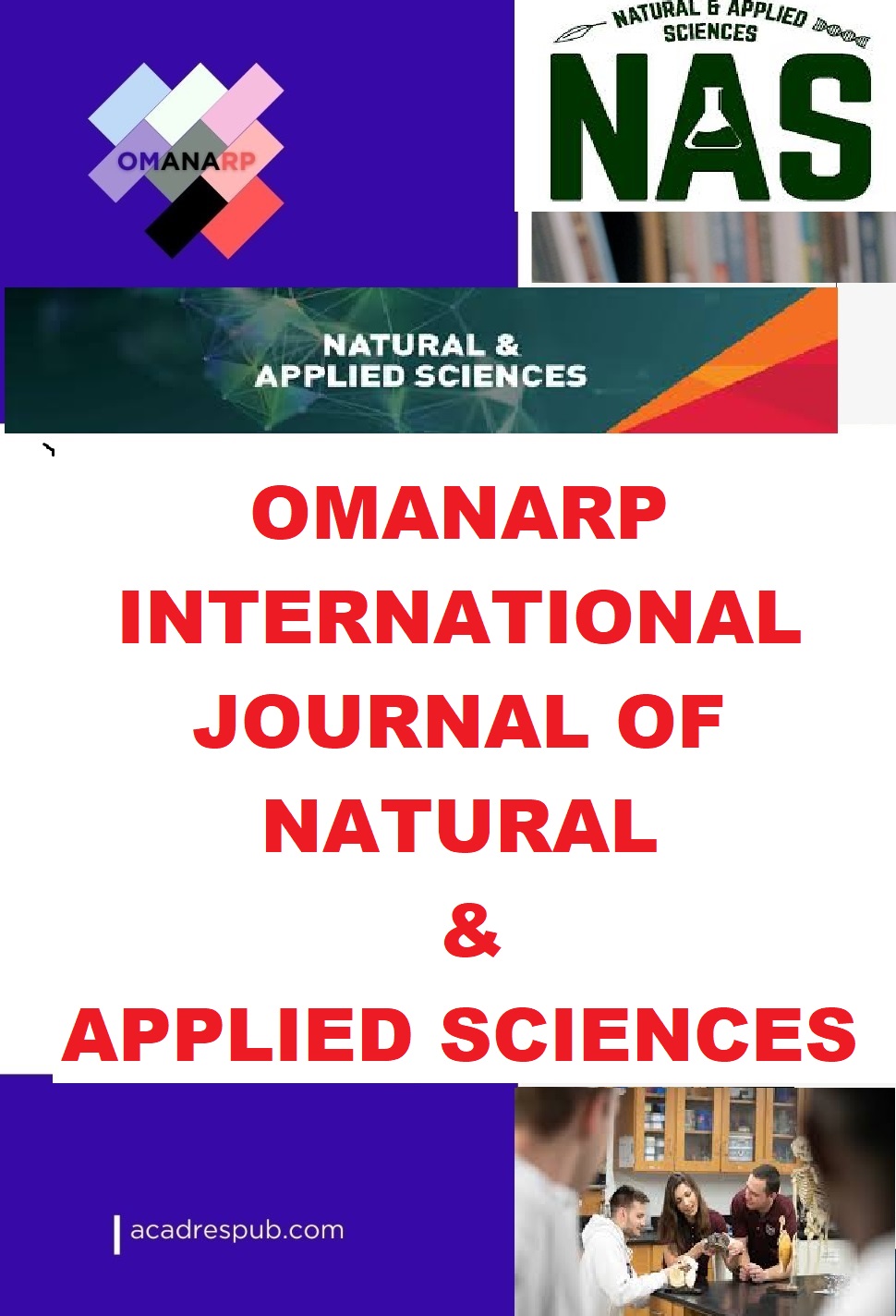